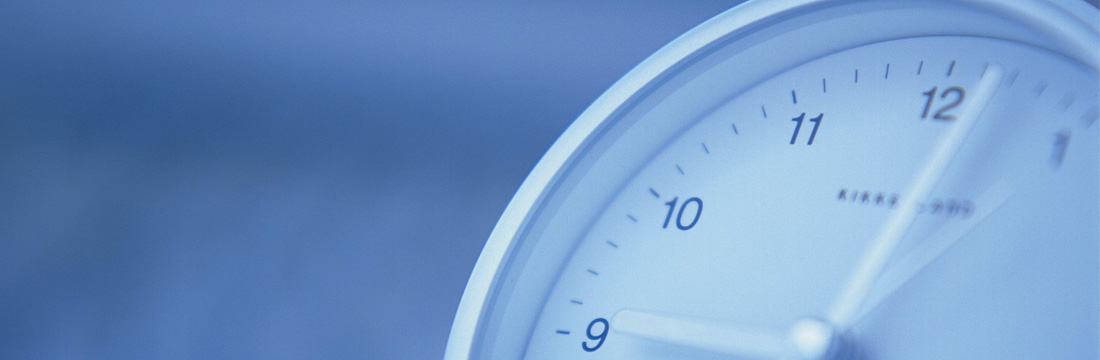
徐宗本
长期从事智能信息处理基础理论研究。
个性化签名
- 姓名:徐宗本
- 目前身份:
- 担任导师情况:
- 学位:
-
学术头衔:
“973”、“863”首席科学家, 博士生导师
- 职称:-
-
学科领域:
应用数学
- 研究兴趣:长期从事智能信息处理基础理论研究。
徐宗本,生于1955年,汉族,教授、博士生导师。1987年在西安交通大学数学系获博士学位,英国strathclyde大学博士后,现任西安交通大学副校长,西安交通大学信息与系统科学研究所所长、国务院学位委员会数学学科评议组成员、信息学部计算机学科评审组成员、教育部数学与统计学教学指导委员会副主任委员、数学类专业教学指导分委员会主任委员、博士生导师,曾任西安交通大学理学院院长,现为国家重点基础研究计划(973)“基于视觉认知的非结构化信息处理基础理论与关键技术”首席科学家。
徐宗本教授长期从事智能信息处理基础理论研究。在数据建模的核心基础,特别是机器学习领域取得系统性创新成果, 并做出了重大贡献。将数据建模从“辅助作图”层次提升到“认知模拟”层次,系统提出了基于视觉认知的数据建模新原理与新方法;发现非欧氏框架下的“类二项式公式”数据建模新工具,奠定了机器学习正则化方法的分析基础,被国外称之为“xu-roach”公式;解决了神经网络系统、模拟进化计算中的一些重要基础问题,相关论文被nature review作为sec代表性文献引用;首次提出用“种群多样度”概念刻画sec假收敛的起因与特征,被相关文献称为是“梁-高-徐度量”。发表学术论文172篇,sci索引114篇, sci引用782次 (他引642次),平均被引用6.86次/篇。研究结果《基于认知与非欧式框架的数据建模基础理论研究》曾获国家自然科学二等奖(2007), 2008年获得我国应用数学最高奖项csiam苏步青数学奖。
-
全讯担保网主页访问
3135
-
关注数
2
-
成果阅读
673
-
成果数
9
上传时间
2009-05-31
徐宗本, zongben xu& hai zhang, †& yao wang & xiangyu chang
sci china ser f-inf sci, jan. 2009, vol. 52. no.1, 1-9,-0001,():
-1年11月30日
in this paper we propose an l1/2 regularizer which has a nonconvex penalty. the l1/2 regularizer is shown to have many promising properties such as unbiasedness, sparsity and oracle properties. a reweighed iterative algorithm is proposed so that the solution of the l1/2 regularizer can be solved through transforming it into the solution of a series of l1 regularizers. the solution of the l1/2 regularizer is more sparse than that of the l1 regularizer, while solving the l1/2 regularizer ismuch simpler than solving the l0 regularizer. the experiments show that the l1/2 regularizer is very useful and efficient, and can be taken as a representative of the lp(0
machine learning,, variable selection,, regularizer,, compressed sensing.,
-
243浏览
-
0点赞
-
0收藏
-
1分享
-
140下载
-
引用
上传时间
2009-05-31
徐宗本, bin zou, luoqing li, zongben xu
published online: 07 february 2009,-0001,():
-1年11月30日
the generalization performance is the main concern of machine learning theoretical research. the previous main bounds describing the generalization ability of the empirical risk minimization (erm) algorithm are based on independent and identically distributed (i.i.d.) samples. in order to study the generalization performance of the erm algorithm with dependent observations, we first establish the exponential bound on the rate of relative uniform convergence of the erm algorithm with exponentially strongly mixing observations, and then we obtain the generalization bounds and prove that the erm algorithm with exponentially strongly mixing observations is consistent. the main results obtained in this paper not only extend the previously known results for i.i.d. observations to the case of exponentially strongly mixing observations, but also improve the previous results for strongly mixing samples. because the erm algorithm is usually very time-consuming and overfitting may happen when the complexity of the hypothesis space is high, as an application of our main results we also explore a new strategy to implement the erm algorithm in high complexity hypothesis space.
generalization performance, erm principle, relative uniform convergence, exponentially strongly mixing
-
60浏览
-
0点赞
-
0收藏
-
1分享
-
94下载
-
引用
上传时间
2009-05-31
徐宗本, xu zongben & wang jianjun
science in china series f: information sciences 2006 vol. 49 no.4 446-460,-0001,():
-1年11月30日
for the nearly exponential type of feedforward neural networks (nefnns), it is revealed the essential order of their approximation. it is proven that for any continuous function defined on a compact set of rd, there exists a three-layer nefnns with fixed number of hidden neurons that attain the essential order. when the function to be approximated belongs to the α-lipschitz family (0<α≤2), the essential order of approximation is shown to be o(n-α) where n is any integer not less than the reciprocal of the predetermined approximation error. the upper bound and lower bound estimations on approximation precision of the nefnns are provided. the obtained results not only characterize the intrinsic property of approximation of the nefnns, but also uncover the implicit relationship between the precision (speed) and the number of hidden neurons of the nefnns.
nearly exponential type neural networks,, the essential order of approximation,, the modulus of smoothness of a multivariate function.,
-
65浏览
-
0点赞
-
0收藏
-
1分享
-
87下载
-
引用
上传时间
2009-05-31
徐宗本, zong-ben xua, hong qiaob, jigen penga, bo zhangc, *
neural networks 17(2004)73-85,-0001,():
-1年11月30日
the neuron state modeling and the local field modeling provides two fundamental modeling approaches to neural network research, based on which a neural network system can be called either as a static neural network model or as a local field neural network model. these two models are theoretically compared in terms of their trajectory transformation property, equilibrium correspondence property, nontrivial attractive manifold property, global convergence as well as stability in many different senses. the comparison reveals an important stability invariance property of the two models in the sense that the stability (in any sense) of the static model is equivalent to that of a subsystem deduced from the local field model when restricted to a specific manifold. such stability invariance property lays a sound theoretical foundation of validity of a useful, cross-fertilization type stability analysis methodology for various neural network models.
static neural network modeling, local field neural network modeling, recurrent neural networks, stability analysis, asymptotic stability, exponential stability, global convergence, globally attractive
-
44浏览
-
0点赞
-
0收藏
-
1分享
-
65下载
-
引用
上传时间
2009-05-31
徐宗本, kwong-sak leung, senior member, ieee, qi-hong duan, zong-ben xu, and c. k. wong, fellow
ieee transactions on evolutionary computation, vol. 5, no.1, february 2001,-0001,():
-1年11月30日
there have been various algorithms designed for simulating natural evolution. this paper proposes a new simulated evolutionary computation model called the abstract evolutionary algorithm (aea), which unifies most of the currently known evolutionary algorithms and describes the evolution as an abstract stochastic process composed of two fundamental operators: selection and evolution operators. by axiomatically characterizing the properties of the fundamental selection and evolution operators, several general convergence theorems and convergence rate estimations for the aea are established. the established theorems are applied to a series of known evolutionary algorithms, directly yielding new convergence conditions and convergence rate estimations of various specific genetic algorithms and evolutionary strategies. the present work provides a significant step toward the establishment of a unified theory of simulated evolutionary computation.
aggregating and scattering rate,, evolutionary strategy,, genetic algorithm,, selection intensity,, selection pressure,, stochastic process.,
-
52浏览
-
0点赞
-
0收藏
-
1分享
-
116下载
-
引用
上传时间
2009-05-31
徐宗本, hong qiao, jigen peng, and zong-ben xu
ieee transactions on neural networks, vol. 12, no.2, march 2001,-0001,():
-1年11月30日
in this paper, a new concept called nonlinear measure is introduced to quantify stability of nonlinear systems in the way similar to the matrix measure for stability of linear systems. based on the new concept, a novel approach for stability analysis of neural networks is developed. with this approach, a series of new sufficient conditions for global and local exponential stability of hopfield type neural networks is presented, which generalizes those existing results. by means of the introduced nonlinear measure, the exponential convergence rate of the neural networks to stable equilibrium point is estimated, and, for local stability, the attraction region of the stable equilibrium point is characterized. the developed approach can be generalized to stability analysis of other general nonlinear systems.
global exponential stability,, hopfield-type neural networks,, local exponential stability,, matrix measure,, nonlinear measures.,
-
49浏览
-
0点赞
-
0收藏
-
1分享
-
38下载
-
引用
上传时间
2009-05-31
徐宗本, zong-ben xu, guo-qing hu and chung-ping kwong
neural networks vol. 9 no.3 pp. 483-501, 1996,-0001,():
-1年11月30日
the hopfield-type networks with asymmetric interconnections are studied from the standpoint of taking them as computational models. two fundamental properties, feasibility and reliability, of the networks related to their use are established with a newly-developed convergence principle and a classification theory on energy functions. the convergence principle generalizes that previously known for symmetric networks and underlies the feasibility. the classification theory, which categorizes the traditional energy functions into regular, normal and complete ones according to their roles played in connection with the corresponding networks, implies that the reliability and high efficiency of the networks can follow respectively from the regularity and the normality of the corresponding energy functions. the theories developed have been applied to solve a classical np-hard graph theory problem: finding the maximal independent set of a graph. simulations demonstrate that the algorithms deduced from the asymmetric theories outperform those deduced from the symmetric theory.
asymmetric hopfield-type networks,, convergence principle,, classification theory on energy functions,, regular and normal correspondence,, maximal independent set problem,, combinatorial optimization.,
-
43浏览
-
0点赞
-
0收藏
-
1分享
-
17下载
-
引用
上传时间
2009-05-31
徐宗本, zong-ben xu and chung-ping kwong
neural networks vol. 9 no.6 pp. 999-1016, 1996,-0001,():
-1年11月30日
a decomposition principle is developed for systematic determination of the dimensionality and the connections of hopfield-type associative memory networks. given a set of high dimensional prototype vectors of given memory objects, we develop decomposition algorithms to extract a set o flower dimensional key features of the pattern vectors. every key feature can be used to build an associative memory with the lowest complexity, and more than one key feature can be simultaneously used to build networks with higher recognition accuracy. in the latter case, we further propose a "decomposed neural network" based on a new encoding scheme to reduce the network complexity. in contrast to the original hopfield network, the decomposed networks not only increase the network's storage capacity, but also reduce the network's connection complexity from quadratic to linear growth with the network dimension. both theoretical analysis and simulation results demonstrate that the proposed principle is powerful.
decomposition principle,, hopfield-type networks,, interpolation operator,, best approximation projection,, associative memories,, elementary matrix transformation
-
49浏览
-
0点赞
-
0收藏
-
1分享
-
27下载
-
引用
上传时间
2009-05-31
徐宗本, yee leung, jiang-she zhang, and zong-ben xu
ieee transactions on neural networks, vol. 8, no.3, may 1997,-0001,():
-1年11月30日
computing convex hull is one of the central problems账in various applications of computational geometry. in this paper, a convex hull computing neural network (chcnn) is developed to solve the related problems in the n-dimensional spaces. the algorithm is based on a two-layered neural network, topologically similar to art, with a newly developed adaptive training strategy called excited learning. the chcnn provides a parallel on-line and real-time processing of data which, after training, yields two closely related approximations, one from within and one from outside, of the desired convex hull. it is shown that accuracy of the approximate convex hulls obtained is around o[k-1=(n-1)], where k is the number of neurons in the output layer of the chcnn. when k is taken to be sufficiently large, the chcnn can generate any accurate approximate convex hull. we also show that an upper bound exists such that the chcnn will yield the precise convex hull when k is larger than or equal to this bound. a series of simulations and applications is provided to demonstrate the feasibility, effectiveness, and high efficiency of the proposed algorithm.
art-like neural network,, computational geometry,, convex hull computation,, excited learning.,
-
68浏览
-
0点赞
-
0收藏
-
1分享
-
126下载
-
引用